Table Of Content
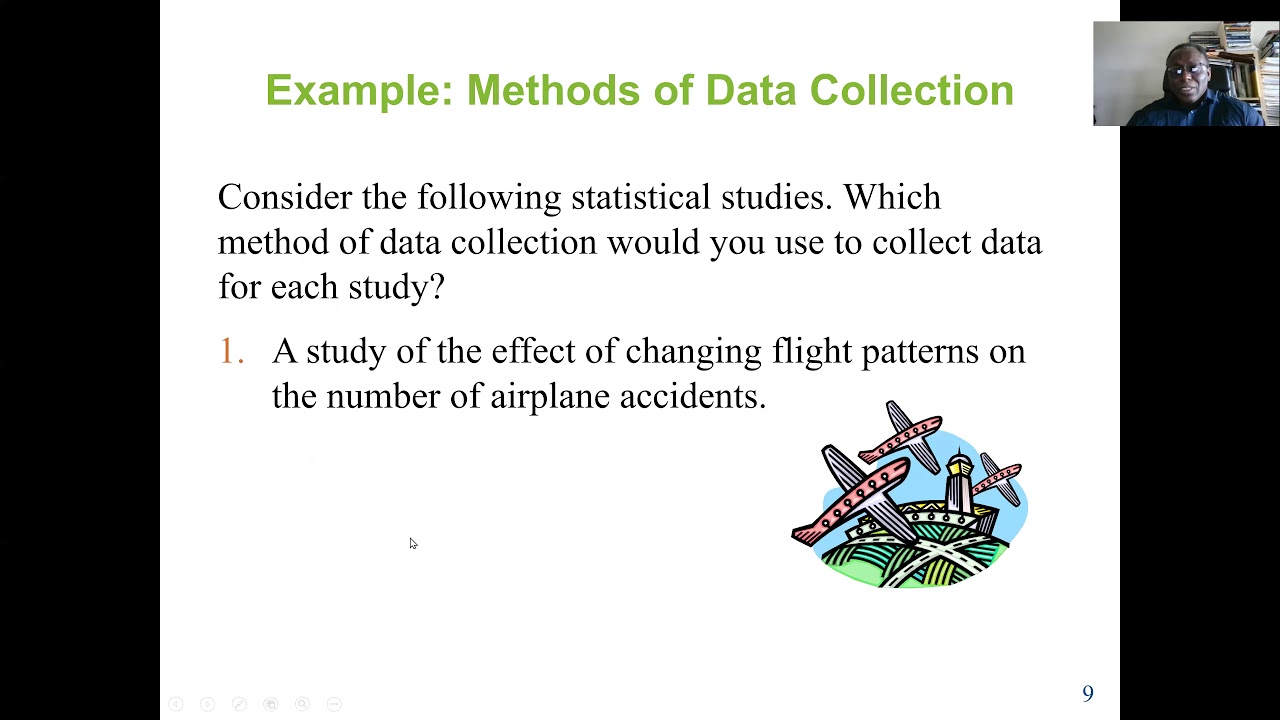
It is particularly useful when studying the impact of an intervention or treatment on a particular outcome. Physiological measures involve measuring participants’ physiological responses, such as heart rate, blood pressure, or brain activity, using specialized equipment. These measures may be invasive or non-invasive, and may be administered in a laboratory or clinical setting. Behavioral measures involve measuring participants’ behavior directly, such as through reaction time tasks or performance tests. These measures may be administered using specialized equipment or software.
Challenging current scientific practice: how a shift in research methodology could reduce animal use Lab Animal - Nature.com
Challenging current scientific practice: how a shift in research methodology could reduce animal use Lab Animal.
Posted: Wed, 03 Jan 2024 08:00:00 GMT [source]
Book traversal links for Lesson 1: Introduction to Design of Experiments
If the variation in random errors is relatively small compared to the total variation in the response, we would have evidence for treatment effect. In a between-subjects design, every participant experiences only one condition, and researchers assess group differences between participants in various conditions. Overall, the purpose of experimental design is to provide a rigorous, systematic, and scientific method for testing hypotheses and establishing cause-and-effect relationships between variables. Experimental design is a powerful tool for advancing scientific knowledge and informing evidence-based practice in various fields, including psychology, biology, medicine, engineering, and social sciences. Computerized measures involve using software or computer programs to collect data on participants’ behavior or responses.
Conducting an Experiment in Psychology - Verywell Mind
Conducting an Experiment in Psychology.
Posted: Mon, 30 Oct 2023 07:00:00 GMT [source]
Dept. Psychological Sciences, Purdue University Dept. Psychological Sciences, West Lafayette, USA
In a blocked experiment, heterogenous experimental units (with known sources of heterogenity) are divided into homogenous subgroups, called blocks, and separate randomized experiments are conducted within each block. It should be kept in mind that counterbalancing is not a satisfactory solution if there are complex dependencies between which treatment precedes which and the dependent variable. In these cases, it is usually better to use a between-subjects design than a within-subjects design. A confounding variable is related to both the supposed cause and the supposed effect of the study. It can be difficult to separate the true effect of the independent variable from the effect of the confounding variable.
Step 5: Measure your dependent variable
Self-report measures involve asking participants to report their thoughts, feelings, or behaviors using questionnaires, surveys, or interviews. Replication involves conducting the same experiment with different samples or under different conditions to increase the reliability and validity of the results. Blinding involves keeping participants, researchers, or both unaware of which treatment group participants are in, in order to reduce the risk of bias in the results. In this design, each participant is exposed to all of the different treatments or conditions, either in a random order or in a predetermined order. For example, if it is surmised that a new medicine reduces the effects of illness from 72 hours to 71 hours, this would not be considered statistically significant.
6 Randomization
A double-blind model is considered the best model for clinical trials as it eliminates the possibility of bias on the part of the researcher and the possibility of producing a placebo effect from the subject. Older women are less likely to be smokers, and older women are more likely to die. Because age is a variable that influences the explanatory and response variable, it is considered a confounding variable. If you have a treatment group and a control group then, in this case, you probably only have one factor with two levels.
The quality of your sampling and the design of your experiment can have an effect upon the outcome and determine whether your hypothesis is rejected or not. Therefore it is important to have an appropriate and properly designed experiment. Within-subjects or repeated measures can also refer to an experimental design where an effect emerges over time, and individual responses are measured over time in order to measure this effect as it emerges. In a between-subjects design (also known as an independent measures design or classic ANOVA design), individuals receive only one of the possible levels of an experimental treatment.
However, this ignores the possibility of chance differences between the groups. That is, by chance, the raters in one condition might have, on average, been more lenient than the raters in the other condition. Randomly assigning subjects to treatments ensures that all differences between conditions are chance differences; it does not ensure there will be no differences. The key question, then, is how to distinguish real differences from chance differences. The inferential statistics applicable to testing the difference between the means of the two conditions can be found here.
There are even laws in the federal code about the use of research data. Clearly, it is never acceptable to falsify data the way this researcher did. Notice that the lurking variable, age, can also be a contributing factor. While there is a correlation between smoking and mortality, and also a correlation between smoking and age, we aren’t 100% sure that they are the cause of the mortality rate in women. The issue of measurement bias arises due to unrecognizable differences in the evaluation process. In an investigation of the effects of education on income, the factor being studied is education level (qualitative but ordinal).
Step 1: Define your variables
An experimental unit is a single object or individual to be measured. In a controlled experiment, the researchers, or investigators, decide which subjects are assigned to a control group and which subjects are assigned to a treatment group. In doing so, we ensure that the control and treatment groups are as similar as possible, and limit possible confounding influences such as lurking variables. A replicated experiment that is repeated on many different subjects helps reduce the chance of variation on the results. And randomization means we randomly assign subjects into control and treatment groups. Regression analysis involves identifying the relationship between a dependent variable and one or more independent variables.
For experimental designs involving multiple factors, a test for the significance of each individual factor as well as interaction effects caused by one or more factors acting jointly can be made. Further discussion of the analysis of variance procedure is contained in the subsequent section. The purpose of an experiment is to investigate the relationship between two variables. When one variable causes change in another, we call the first variable the explanatory variable. In a randomized experiment, the researcher manipulates values of the explanatory variable and measures the resulting changes in the response variable. The different values of the explanatory variable are called treatments.
The same is true for intervening variables (a variable in between the supposed cause (X) and the effect (Y)), and anteceding variables (a variable prior to the supposed cause (X) that is the true cause). When a third variable is involved and has not been controlled for, the relation is said to be a zero order relationship. In most practical applications of experimental research designs there are several causes (X1, X2, X3). This involves randomly assigning participants to different groups or treatments to ensure that any observed differences between groups are due to the treatment and not to other factors. Is one brand of fertilizer more effective at growing roses than another? In this module, you will learn important aspects of experimental design.
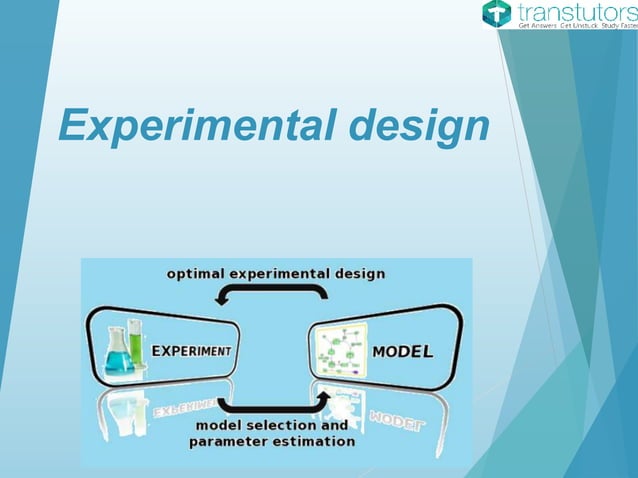
Experimental designs are a set of procedures that you plan in order to examine the relationship between variables that interest you. How you apply your experimental treatments to your test subjects is crucial for obtaining valid and reliable results. Then you need to think about possible extraneous and confounding variables and consider how you might control them in your experiment. To translate your research question into an experimental hypothesis, you need to define the main variables and make predictions about how they are related. SEM is a statistical technique used to model complex relationships between variables. Factor analysis is used to identify underlying factors or dimensions in a set of variables.
Designs can contain combinations of between-subject and within-subject variables. For example, the "Weapons and Aggression" case study has one between-subject variable (gender) and two within-subject variables (the type of priming word and the type of word to be responded to). In this course we will pretty much cover the textbook - all of the concepts and designs included. I think we will have plenty of examples to look at and experience to draw from.
There are multiple approaches for determining the set of design points (unique combinations of the settings of the independent variables) to be used in the experiment. The use of a completely randomized design will yield less precise results when factors not accounted for by the experimenter affect the response variable. Consider, for example, an experiment designed to study the effect of two different gasoline additives on the fuel efficiency, measured in miles per gallon (mpg), of full-size automobiles produced by three manufacturers. Suppose that 30 automobiles, 10 from each manufacturer, were available for the experiment. In a completely randomized design the two gasoline additives (treatments) would be randomly assigned to the 30 automobiles, with each additive being assigned to 15 different cars. Suppose that manufacturer 1 has developed an engine that gives its full-size cars a higher fuel efficiency than those produced by manufacturers 2 and 3.
These measures may include reaction time tasks, cognitive tests, or other types of computer-based assessments. The use of a control group is an important experimental design method that involves having a group of participants that do not receive the treatment or intervention being studied. The control group is used as a baseline to compare the effects of the treatment group. This design involves dividing participants into blocks based on a specific characteristic, such as age or gender, and then randomly assigning participants within each block to one of two or more treatment groups.
Double-blind study is where neither the individual nor the researcher knows who is getting which treatment or who is getting the treatment and who is getting the placebo. This is important so that there can be no bias created by either the individual or the researcher. Repetition of an experiment on more than one subject so you can make sure that the sample is large enough to distinguish true effects from random effects. It is also the ability for someone else to duplicate the results of the experiment.
No comments:
Post a Comment